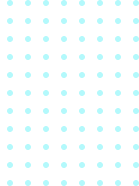
AB Testing Statistics: Understanding the Numbers
AB Testing Statistics: Understanding the Numbers
03-02-2025 (Last modified: 03-02-2025)
Becky Halls
A/B testing is a fundamental tool for marketers and businesses looking to improve conversion rates, optimize user experience, and make data-driven decisions. However, without a solid grasp of AB testing statistics, interpreting results can lead to misleading conclusions.
This guide will break down the essential statistical concepts behind A/B testing, key metrics to track, and best practices to ensure your tests produce reliable insights.
Why AB Testing Statistics Matter
A/B testing isn’t just about seeing which version performs better; it’s about using statistical analysis to ensure that differences in performance are meaningful and not due to chance. Without a proper understanding of AB testing statistics, businesses risk making decisions based on inaccurate or insignificant data.
By leveraging statistical principles, you can:
- Avoid false positives or negatives
- Ensure results are statistically significant
- Make confident, data-driven decisions
- Optimize marketing strategies based on real user behavior
Key Statistical Concepts in A/B Testing
To accurately interpret AB testing statistics, you need to understand the following metrics and principles:
1. Sample Size
The number of participants included in an A/B test significantly impacts the reliability of results. If your sample size is too small, random variation could skew the outcome, leading to inaccurate conclusions.
Best Practice: Use an A/B testing sample size calculator to determine the number of visitors needed for statistically significant results.
2. Statistical Significance
Statistical significance measures the likelihood that the observed differences in performance are real and not due to random chance. Most A/B tests aim for a 95% confidence level, meaning there’s only a 5% probability that the results are due to chance.
Example: If Version B has a p-value of 0.03, it means there’s only a 3% chance that the results happened randomly—making it statistically significant.
3. Confidence Interval
A confidence interval provides a range within which the true conversion rate is likely to fall. If the confidence interval of Version B overlaps significantly with Version A, the results may not be conclusive.
Example:
- Version A: 3.2% – 4.5% conversion rate
- Version B: 4.1% – 5.3% conversion rate
Since there’s little overlap, Version B is likely the better performer.
4. P-Value
The p-value measures the probability that differences in A/B test results happened by chance. A p-value lower than 0.05 typically indicates statistical significance.
Example: A p-value of 0.02 suggests a 2% probability that the results are random, meaning you can be 98% confident that Version B is actually better.
5. Conversion Rate and Uplift
- Conversion Rate: The percentage of users who take a desired action (e.g., clicking a CTA, purchasing a product).
- Uplift: The percentage increase in conversions between Version A and Version B.
Example:
- Version A: 5% conversion rate
- Version B: 6% conversion rate
- Uplift: ((6-5)/5) * 100 = 20% improvement
6. Type I and Type II Errors
- Type I Error (False Positive): Concluding a change is effective when it isn’t.
- Type II Error (False Negative): Failing to detect a real improvement.
Using A/B testing statistics, you can minimize these errors and ensure that test results are reliable before making major decisions.
Best Practices for Interpreting AB Testing Statistics
Understanding AB testing statistics is only useful if you apply best practices when analyzing results. Here’s how to ensure accuracy and effectiveness:
1. Let Tests Run Long Enough
- Prematurely stopping a test can lead to misleading results.
- Aim for at least two weeks or until statistical significance is reached.
- Consider traffic consistency to avoid fluctuations.
2. Test One Variable at a Time
- Testing multiple variables at once makes it difficult to pinpoint what caused the difference in results.
- For complex experiments, consider multivariate testing instead of A/B testing.
3. Use A/B Testing Tools for Accuracy
Reliable A/B testing tools ensure accurate data collection and analysis:
- PageTest.ai (AI-powered testing and optimization)
- Google Optimize (now discontinued, alternatives include PageTest.ai)
- Optimizely (Enterprise-level testing)
- VWO (Comprehensive testing with behavioral insights)
4. Segment Your Data for Deeper Insights
Different audience segments may react differently to changes. Consider segmenting results by:
- Device type (mobile vs. desktop)
- Traffic source (organic vs. paid)
- New vs. returning users
5. Track Secondary Metrics
While conversion rate is the primary focus, other metrics like bounce rate, time on page, and average order value provide additional insights into user behavior.
6. Document and Iterate
- Keep a record of every test, including hypothesis, results, and insights.
- Use findings to inform future tests and continuously refine your strategy.
Common Mistakes When Interpreting Testing Statistics
Even experienced marketers can make mistakes when analyzing AB testing statistics. Here are some pitfalls to avoid:
1. Running Tests with Insufficient Traffic
If your sample size is too small, results may be misleading. Use an A/B test sample size calculator before launching an experiment.
2. Ending Tests Too Soon
If you stop a test as soon as you see a difference, you might be acting on temporary fluctuations. Always wait for statistical significance.
3. Ignoring External Factors
- Seasonal trends, promotions, and competitor activity can affect results.
- Run tests under stable conditions for the most reliable insights.
4. Not Considering Variability
A test’s outcome can vary across different segments, devices, or times of the day. Always analyze segmented data before drawing conclusions.
Final Thoughts: Making Data-Driven Decisions
Understanding AB testing statistics is essential for running meaningful experiments that drive real improvements. By focusing on statistical significance, sample size, p-values, and confidence intervals, businesses can ensure that test results are reliable and actionable.
Key Takeaways: ✔ Statistical significance ensures reliable A/B testing results. ✔ Large enough sample sizes prevent false conclusions. ✔ Segmenting data provides deeper insights into user behavior. ✔ Using the right tools and best practices leads to better optimization decisions.
By mastering AB testing statistics, you’ll be able to make smarter, data-driven choices that improve conversion rates and overall marketing performance. Start testing today and let the numbers guide you to success!
say hello to easy Content Testing
try PageTest.AI tool for free
Start making the most of your websites traffic and optimize your content and CTAs.
Related Posts
14-03-2025
Becky Halls
Search Engine Optimization for Mobile: A Complete Guide
Mobile search isn’t the future—it’s already here. With over 60% of global web traffic coming from mobile devices, search engine optimization for mobile is no longer optional. Google’s mobile-first indexing means that if your website isn’t optimized for smartphones and tablets, you’re missing out on traffic, rankings, and conversions. This guide will walk you through […]
14-03-2025
Becky Halls
Simple Steps to Build a Complete SEO Strategy
SEO is one of those things everyone knows they should be doing, but very few actually get right. It’s not just about stuffing your content with keywords or getting a few backlinks – it’s a multi-layered approach that requires a mix of technical expertise, content strategy, and ongoing analysis. If you’ve ever wondered how to […]
14-03-2025
Becky Halls
Rank Higher & Convert Faster with Mobile Friendly SEO
In a world where people are glued to their smartphones, having a mobile friendly SEO strategy isn’t optional – it’s essential. With Google prioritizing mobile-first indexing, websites that aren’t optimized for mobile users are missing out on traffic, rankings, and conversions. So, how do you make sure your site is mobile-friendly? In this guide, we’ll […]