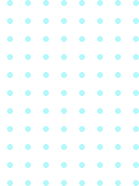
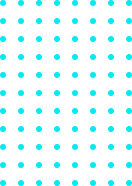
Mastering the A/B Testing Statistical Approach
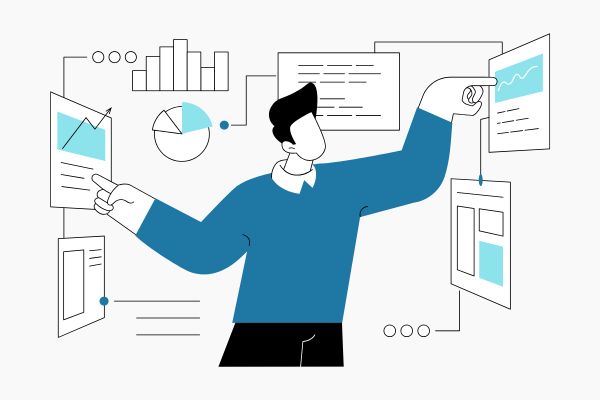
Mastering the A/B Testing Statistical Approach
20-01-2025 (Last modified: 21-05-2025)
Introduction
For those who have embraced the world of A/B testing, the term “statistical significance” is more than just a buzzword; it’s the foundation of meaningful experimentation. Without the proper application of statistical principles, your A/B tests risk producing unreliable or misleading results. This article unpacks the nuances of the A/B testing statistical approach, diving into the key concepts, methodologies, and best practices to ensure your testing efforts are both precise and actionable.
Why the A/B Testing Statistical Approach Matters
At its core, the A/B testing statistical approach is about making decisions based on data rather than intuition. By applying statistical rigor, you can:
- Reduce Risk: Avoid making changes based on random fluctuations.
- Gain Confidence: Ensure that observed improvements are likely to persist over time.
- Optimize Resources: Focus on what truly impacts user behavior rather than chasing noise.
But here’s the catch: A/B testing isn’t just about comparing two versions of a webpage. It’s about ensuring that the results you’re seeing are statistically significant and not due to chance. That’s where statistical principles come in.
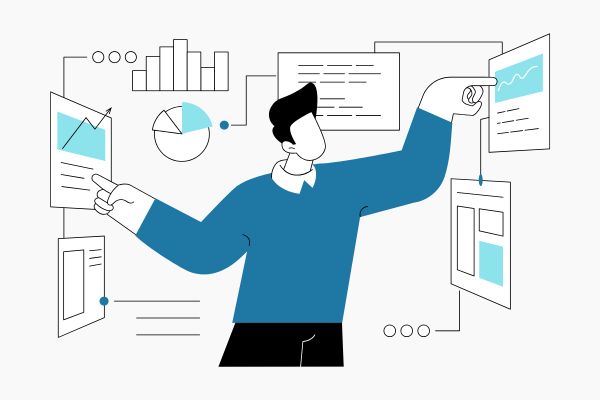
Key Concepts in the A/B Testing Statistical Approach
1. Statistical Significance
Statistical significance indicates whether the difference between Version A and Version B is unlikely to have occurred by chance. Typically, a p-value of 0.05 or lower is used as a benchmark, meaning there’s less than a 5% probability that the observed results are due to random chance.
- Example: If Version B’s conversion rate is higher than Version A’s, a statistically significant result means it’s likely that Version B is genuinely better.
2. Sample Size
Small sample sizes can lead to unreliable results. Calculating the required sample size before running your test ensures your results are robust.
- How to Calculate: Use online calculators or tools like PageTest.ai to determine the minimum number of visitors you need based on your expected lift and baseline conversion rate.
3. Power and Confidence Levels
- Power: The probability of detecting a true effect when it exists. A power of 80% is standard in A/B testing.
- Confidence Level: The probability that the observed results reflect reality. A 95% confidence level is typical.
4. Type I and Type II Errors
- Type I Error (False Positive): Concluding that a variation is better when it’s not.
- Type II Error (False Negative): Missing a genuine improvement because the test failed to detect it.
Balancing these errors is key to a reliable A/B testing statistical approach.
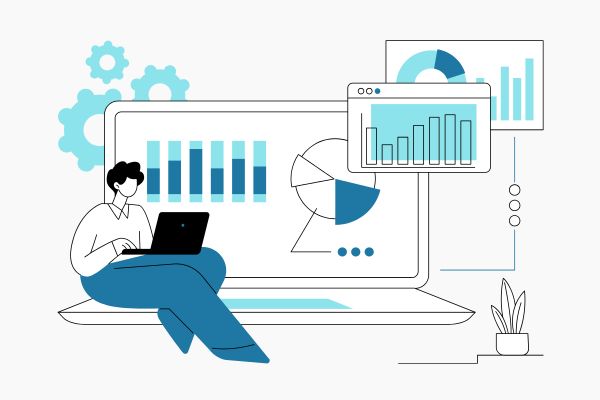
Steps for a Statistically Sound A/B Test
1. Define Your Hypothesis
Every A/B test should begin with a clear hypothesis. It’s not enough to test randomly—you need a specific question to answer.
- Example Hypothesis: “Changing the CTA button color from blue to orange will increase conversions by 10%.”
2. Calculate Sample Size
Determine the number of visitors needed for your test. Factors to consider include:
- Baseline conversion rate.
- Minimum detectable effect (the smallest change you want to detect).
- Confidence level and power.
3. Randomly Assign Traffic
Ensure that visitors are evenly and randomly split between Version A and Version B. This minimizes bias and ensures the reliability of your results.
4. Run the Test for an Appropriate Duration
Stopping your test too early can lead to inaccurate conclusions. Aim to run the test for at least one full business cycle (often a week or two) to account for variations in user behavior.
5. Analyze the Results
Use statistical tools to analyze your data. Calculate the p-value and confidence intervals to determine whether the observed differences are significant.
6. Make Data-Driven Decisions
If your results are statistically significant, implement the winning variation. If not, consider refining your test or exploring other elements.
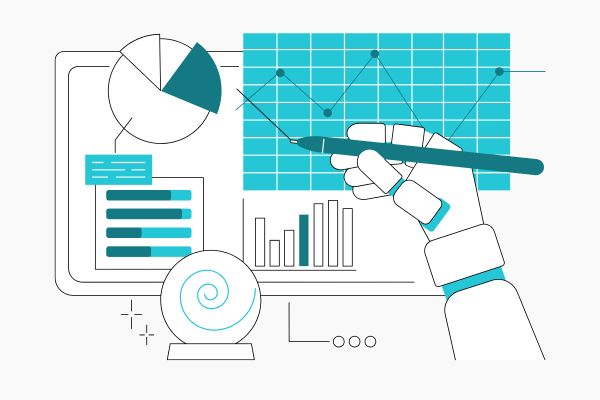
Tools for Advanced Statistical Analysis
Several tools can simplify the A/B testing statistical approach:
- PageTest.ai: Offers AI-powered suggestions and calculates statistical significance for your tests.
- Google Sheets or Excel: Use built-in functions to calculate p-values and confidence intervals.
- R or Python: For more complex statistical modeling and analysis.
Common Pitfalls in the A/B Testing Statistical Approach
1. Running Too Many Tests Simultaneously
Testing multiple elements at once can lead to interactions that skew results. Use multivariate testing if you want to explore multiple variables.
2. Stopping Tests Too Early
Impatient? Stopping a test before reaching the required sample size can lead to misleading conclusions.
- Solution: Commit to running tests for the full duration required to gather statistically significant data.
3. Ignoring Seasonal Effects
Seasonal variations can impact user behavior. Consider running your test across multiple time periods to ensure reliability.
4. Misinterpreting Results
Statistical significance doesn’t guarantee practical significance. Always consider the actual impact of changes, even if they’re statistically valid.
The Role of Bayesian Statistics in A/B Testing
While most A/B testing relies on frequentist statistics, Bayesian statistics offers an alternative approach. Instead of focusing solely on p-values, Bayesian methods calculate the probability that one version is better than the other.
- Benefits:
- Allows for more intuitive decision-making.
- Can incorporate prior knowledge into the analysis.
- Example Tool: Optimizely supports Bayesian analysis, providing actionable probabilities rather than just significance levels.
Pro Tips for Advanced A/B Testers
- Use Segmentation: Analyze results by user segments (e.g., device type, location) to uncover deeper insights.
- Combine Quantitative and Qualitative Data: Pair A/B test results with user feedback for a comprehensive understanding.
- Test Continuously: Optimization is an ongoing process. Use insights from one test to inform future experiments.
- Educate Your Team: Ensure stakeholders understand the principles of the A/B testing statistical approach to foster buy-in and collaboration.
Conclusion: Mastering the A/B Testing Statistical Approach
A/B testing is both an art and a science. By embracing the A/B testing statistical approach, you can ensure your experiments are not only effective but also reliable. From defining hypotheses to analyzing results, every step should be guided by data and statistical rigor. With practice and the right tools, you’ll transform your optimization efforts into a powerful, data-driven machine that delivers real, measurable results.
Q&A: Statistical Insights in A/B Testing
- What is the statistical approach to A/B testing?
It’s the process of using statistical methods to determine whether the results of your test—like increased conversions—are real or just due to random chance. This involves concepts like p-values, confidence levels, and sample size calculations. - Why is statistical significance important in A/B testing?
It tells you whether the observed difference between your test variations is likely to be real or just a fluke. A result is typically considered statistically significant if the p-value is 0.05 or lower. - How do you calculate the right sample size for an A/B test?
Sample size depends on your baseline conversion rate, the minimum effect you want to detect, and your desired confidence and power levels. Use tools like PageTest.ai or online calculators to figure this out before you start testing. - What’s the difference between a Type I and Type II error?
A Type I error means falsely thinking a change worked when it didn’t (false positive), while a Type II error means missing a real improvement (false negative). A solid testing approach balances the risk of both. - What is test power in the context of A/B testing?
Test power is the probability of detecting a true difference when one exists. An 80% power level is standard, meaning there’s a good chance your test will catch real improvements. - Can you stop an A/B test as soon as you see a result?
Nope. Ending a test too early can give you misleading results. Always run your test until it reaches the required sample size and duration for statistical significance. - How long should an A/B test run to be valid?
Ideally, tests should run for at least a full business cycle (like 7–14 days) to account for day-to-day behavioral changes. Cutting it short can compromise your results. - What tools can help with A/B testing statistical analysis?
You can use PageTest.ai for automated significance calculations, or dive deeper with tools like Excel, Google Sheets, R, or Python for custom analysis. - What is Bayesian A/B testing?
Bayesian testing provides a probability that one version is better than the other, rather than relying solely on p-values. It’s a more intuitive, flexible method supported by tools like Optimizely. - How can you make your A/B testing results more reliable?
Define clear hypotheses, calculate the right sample size, segment your audience, avoid testing too many variables at once, and always let your test run its full course. Pair stats with user feedback for the full picture.
say hello to easy Content Testing
try PageTest.AI tool for free
Start making the most of your websites traffic and optimize your content and CTAs.
Related Posts
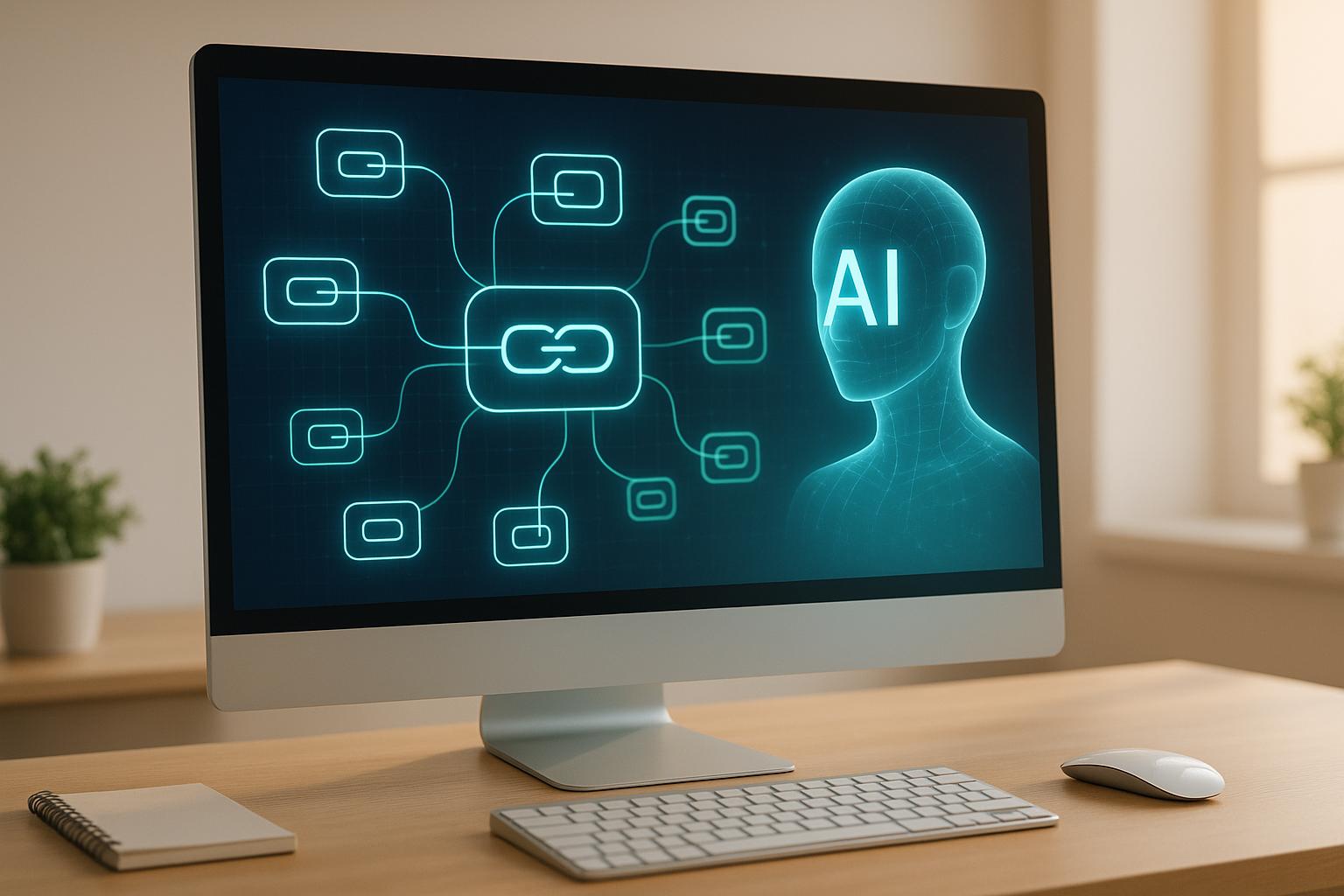
03-07-2025
Ian Naylor
How AI Improves Backlink Quality Checks
Explore how AI enhances backlink quality checks with real-time monitoring, predictive insights, and automated outreach, revolutionizing SEO strategies.
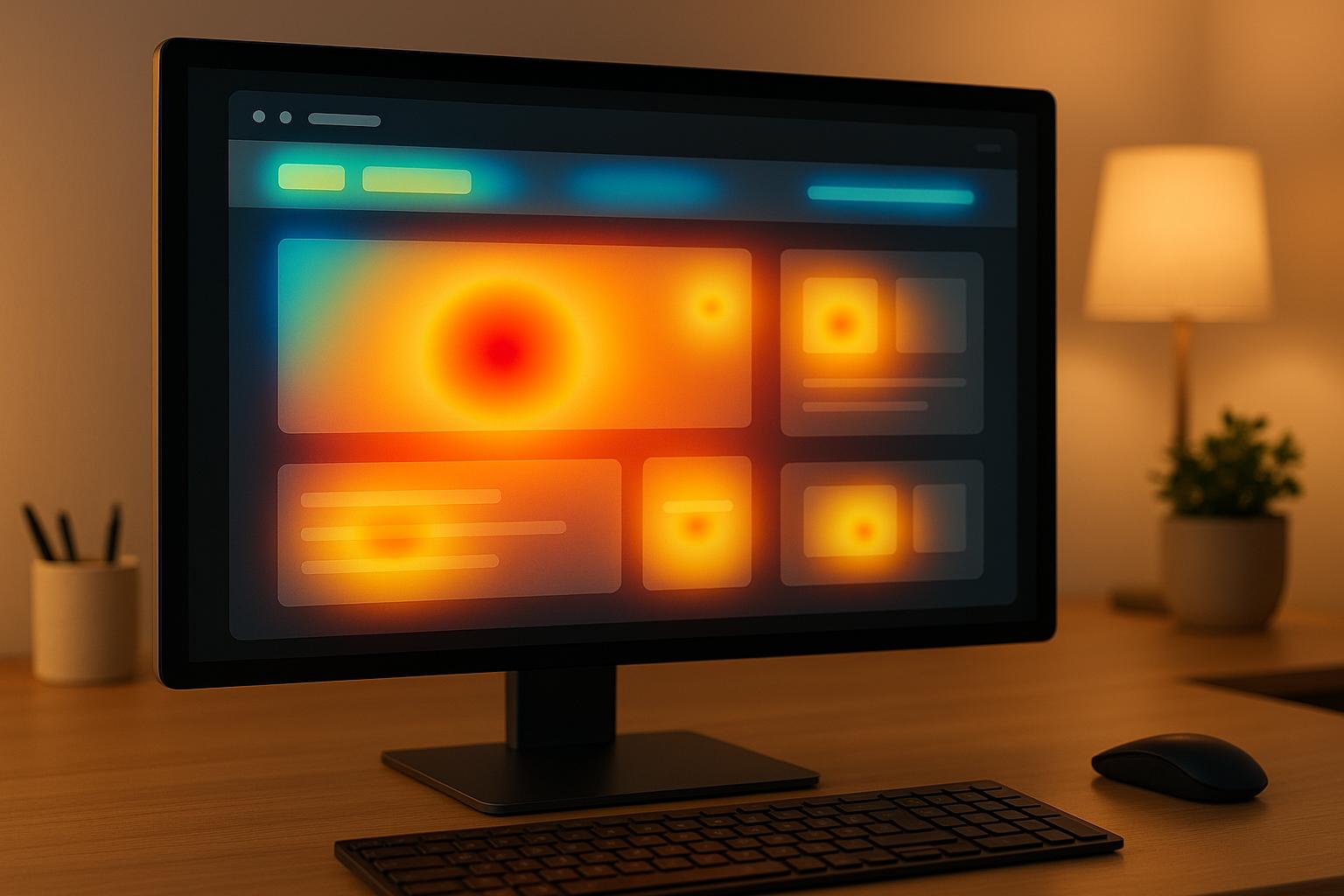
02-07-2025
Ian Naylor
Heatmaps and Click Tracking for CRO
Utilize heatmaps and click tracking to enhance user experience, identify conversion barriers, and boost website performance effectively.
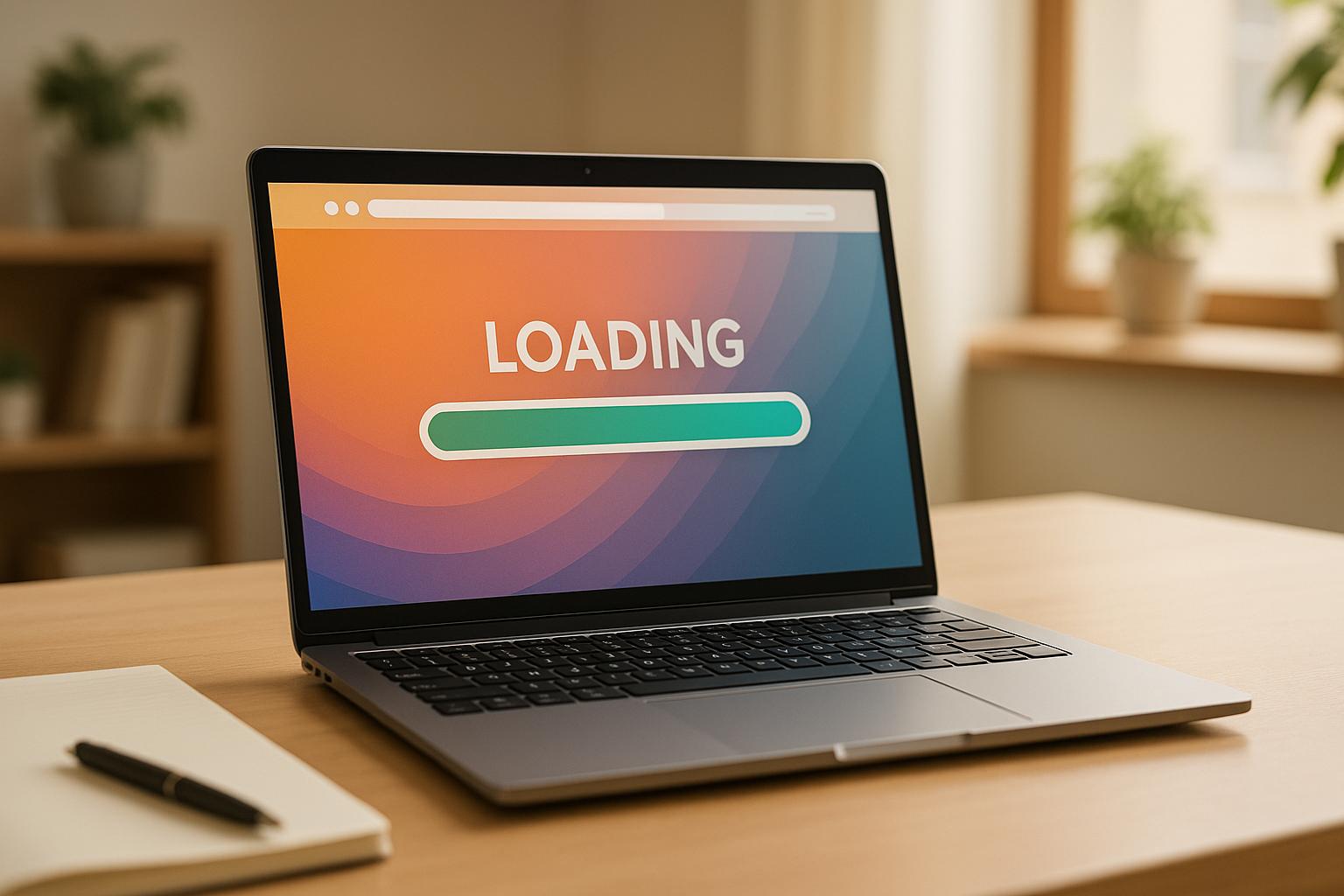
01-07-2025
Ian Naylor
Page Load Time vs. Conversion Rates
Website load times significantly impact user engagement and conversion rates, revealing critical strategies for optimizing performance and boosting revenue.